Perhaps the greatest challenge in responding to the coronavirus pandemic is that governments are being forced to make potentially life and death decisions based on imperfect information. Officials across the globe are relying on statistical models to make critical policy choices, such as how to best direct supplies and expertise, allocate funding, and enforce social distancing rules. One prominent example is the March 16th epidemiological model from Imperial College London that projected, absent aggressive intervention, more than 2 million American and half a million British deaths from COVID-19. This alarming projection prompted the British government to reverse its herd immunity policy, and immediately enforce strict public quarantines. The same model later generated criticism from many quarters when its author amended it to project significantly fewer deaths. Were the authors of the Imperial College London model (and many others like it) wrong?

We believe that the public confusion stemming from these models reflects a common misunderstanding of statistical modeling and its purpose, a challenge which we often see in our work with businesses seeking to employ similar models in their operations. Models are most valuable when utilized to describe a range of possibilities, and the range of possibilities will always be highly sensitive to any reactions. In other words, models impact behavior, which impacts the model, which further impacts behavior - and so on - until the model is inevitably changed. This dynamic process requires constant refinement in order to realize maximum value.
Maintaining this dynamic process is all the more critical in the high-pressure context of a public health crisis. Recent pandemics reflect the difficulties modelers face in these situations. Governments and public health care organizations, such as the World Health Organization (WHO), were similarly challenged by a lack of real-time data in 2009 while modeling the impact of another novel influenza strain: H1N1 ("Swine Flu"). These organizations used similar models to inform policymakers and, as now, faced harsh criticism for the perceived inaccuracy of these models. Then, as now, the scarcity of timely and reliable data led many modelers to make assumptions that limited the models' ability to forecast the outcomes of H1N1.
Confidence Levels
Models can be impacted by the quality and availability of data, the assumptions underlying the data, and the confidence levels in both. For example, the COVID-19 epidemiological models rely on tried and true statistical methods, but the unavailability of data and difficulty in predicting public policies to limit the spread of the virus led to large confidence intervals - or range of potentially correct values - in predicted infection rates. In the Imperial College London study, the authors predicted the number of infections in eleven European countries. The ranges of infection rates between countries varied, sometimes wildly, with Spain's infection rates predicted to be from 3.7% to 41%. These predictions relied on assumptions rooted in the impact of COVID-19 in Italy and China, each of which has its own limitations.
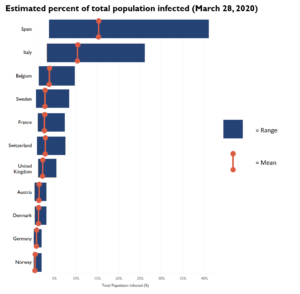
Data Quality Factors
What leads to these issues in predicting the impact of COVID-19 on populations? Most are related to the quality of the underlying data itself, which is impacted by several factors:
1. Testing is focused on severe cases and deaths
In many countries, testing is still limited to hospitals and urgent care facilities which will naturally skew the data toward severe cases and deaths rather than mild cases, which don't present at a hospital. An estimated 81% of COVID-19 cases have mild symptoms and will go undetected. This will be a major source of bias in any estimates using this data.
2. Not enough tests are being administered
Low rates of testing will lead to underreporting of cases and large margins of error in any estimates using this data. By late March, the U.S. had administered around 314 tests per 1 million people: South Korea had conducted 6,148 by that point.
3. Some of the tests are unreliable
The most common type of COVID-19 test, polymerase chain reaction (PCR) may produce false negatives in about 30% of cases, failing to identify evidence of the disease. Testing in the early stages of infections can also be unreliable as the patient may not excrete a detectable amount of virus given the maximum incubation period is currently assumed to be 14 days.
4. Inconsistent test reporting
Some countries report the number of COVID-19 tests administered, other countries report the number of patients tested. Some countries have inconsistent approaches among regions, and in others it is unclear what approach was taken. This further skews the data, as at least some percentage of infected patients are being tested multiple times.
5. Inconsistent death reporting
Estimates from English hospitals show that death counts are reported with a delay of around 2-3 days generally, but in some cases up to two weeks. In the U.S., it has been reported that many deaths related to COVID-19 have not been reported in official statistics, due to the lack of available testing early on.
6. Uncertainty around cause of death
In the U.S. and countries such as Italy, anyone who dies with COVID-19, regardless of any underlying health condition, is currently being counted as a death from COVID-19. It will not be clear how many of those deaths would have occurred anyway if the patients had not contracted COVID-19, given that many deaths are of patients with underlying health conditions.
Organizational Lessons
Statistical Modeling Is Dynamic
Statistical modeling is not a crystal ball; in many cases, it isn't even a prediction. These models should be used to describe a range of possibilities, and the range of possibilities will shift as your organization reacts to new information. This doesn't mean that the modeling process is wrong; it just means that it's a process.
Data Quality Is Paramount
It's impossible for organizations to model potential outcomes without quality data from confirmed sources. In the case of the coronavirus response, we see this play out in a number of ways, particularly with the unknown number of infected. If governments were able to deploy testing at a larger scale and determine this number with confidence, it could completely change the world's approach to the pandemic. This demonstrates how even a single key variable can impact the direction of a model, and resulting policy choices.
Communicate Your Assumptions, and Revisit Them Often
Every model makes assumptions. The author's job is to ensure not only that there is a clear differentiation between the evidence and the assumptions, but also that the audience can understand how the model's projections will change as those assumptions change. When FRA develops models for our clients, we ingrain a process whereby all underlying assumptions are clearly laid out and debated, in order for key decision makers to come to a consensus. When statistical models are perceived to have failed, it is often because one or more key assumptions were not adjusted to reflect new data. Therefore, one of the critical exercises in developing any dynamic model is the process of ensuring an effective feedback loop to stress test the assumptions, and refresh the model with new (often more reliable) information. In the case of both COVID-19 and the Swine Flu, the key assumption many models made early on was that the number of infected roughly correlated to the number of positive tests. We know now that is not the case, which accounts for the significant downward adjustment to the number of predicted deaths in both sets of models.
Utilize Data Analytics to Drive Policy
Modeling is a good way to understand the range of potential outcomes of a course of action, but organizations must dig deeper into the data in order to make use of the models. For example, as outcome data on COVID-19 patients becomes more widely available, policy makers are better able to identify risk factors and vulnerable segments of the population. This is likely to be extremely valuable as decisions are made regarding how to prioritize resources, where and when to relax quarantine rules, etc. Similarly, businesses must take a comprehensive look at their data and tease out trends and outliers, in order to discern valuable business intelligence and drive policy.